Abstract
Optical computing systems provide high-speed and low-energy data processing but face deficiencies in computationally demanding training and simulation-to-reality gaps. We propose a gradient-based model-free optimization (G-MFO) method based on a Monte Carlo gradient estimation algorithm for computationally efficient in situ training of optical computing systems. This approach treats an optical computing system as a black box and back-propagates the loss directly to the optical computing weights' probability distributions, circumventing the need for a computationally heavy and biased system simulation. Our experiments on diffractive optical computing systems show that G-MFO outperforms hybrid training on the MNIST and FMNIST datasets. Furthermore, we demonstrate image-free and high-speed classification of cells from their marker-free phase maps. Our method's model-free and high-performance nature, combined with its low demand for computational resources, paves the way for accelerating the transition of optical computing from laboratory demonstrations to practical, real-world applications.
Comparison of training strategies

Results
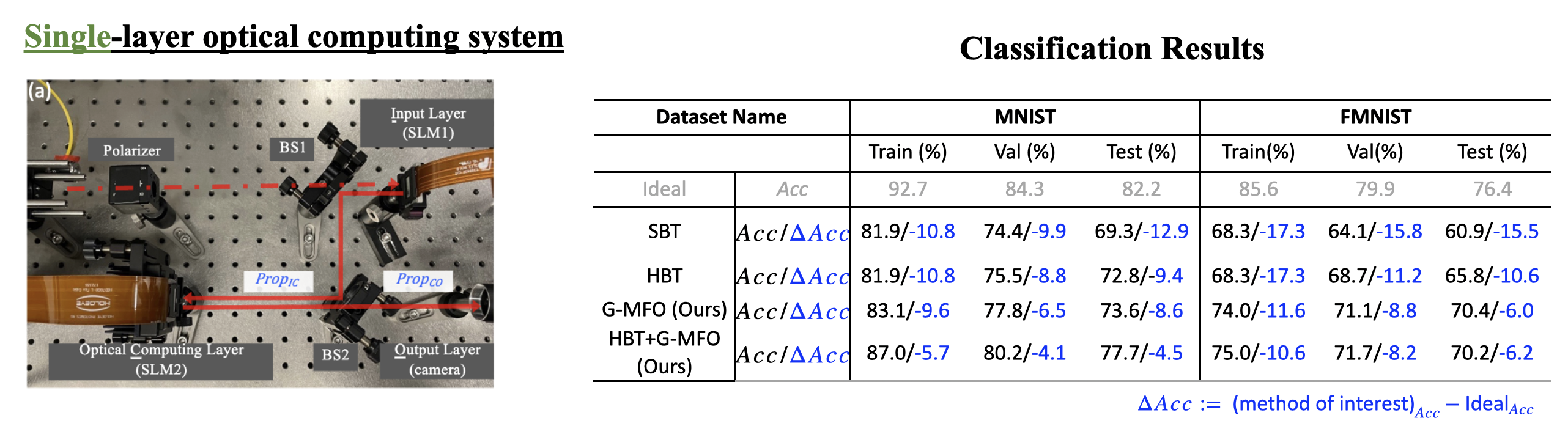
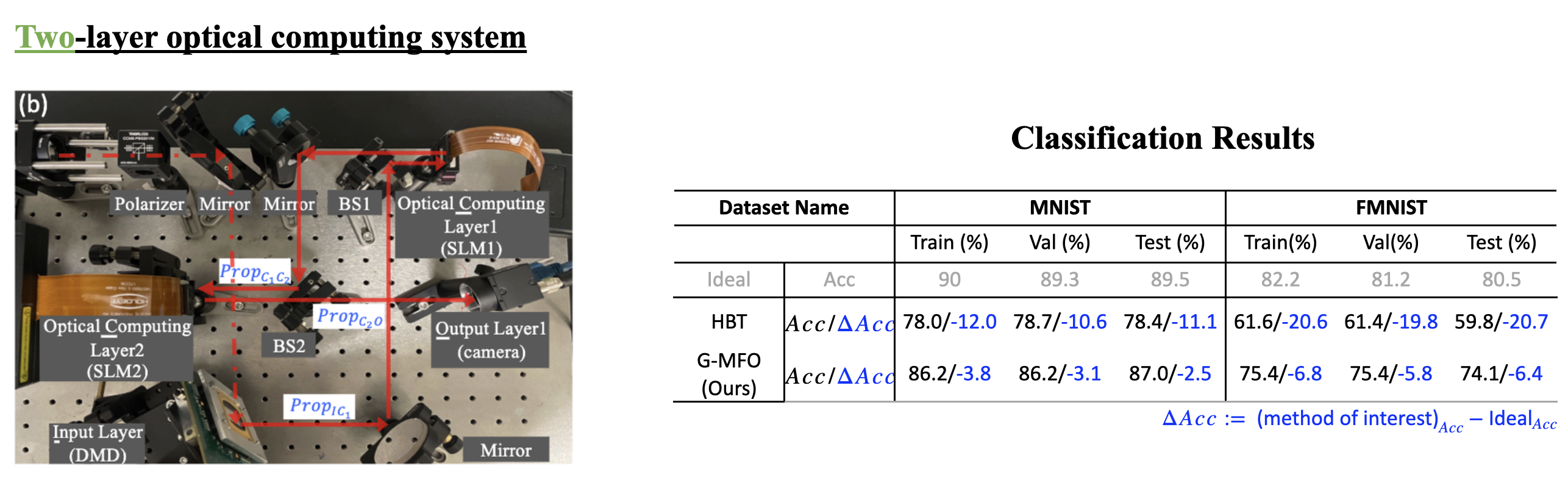
Video
Citation
@article{Zhao2024MFO,
title={High-performance real-world optical computing trained by in situ gradient-based model-free optimization},
author={Zhao, Guangyuan and Shu, Xin and Zhou, Renjie},
journal={IEEE Transactions on Pattern Analysis and Machine Intelligence},
year={2024},
publisher={IEEE}
}